Statistical Methods for Clinical Trials of TB Therapeutics
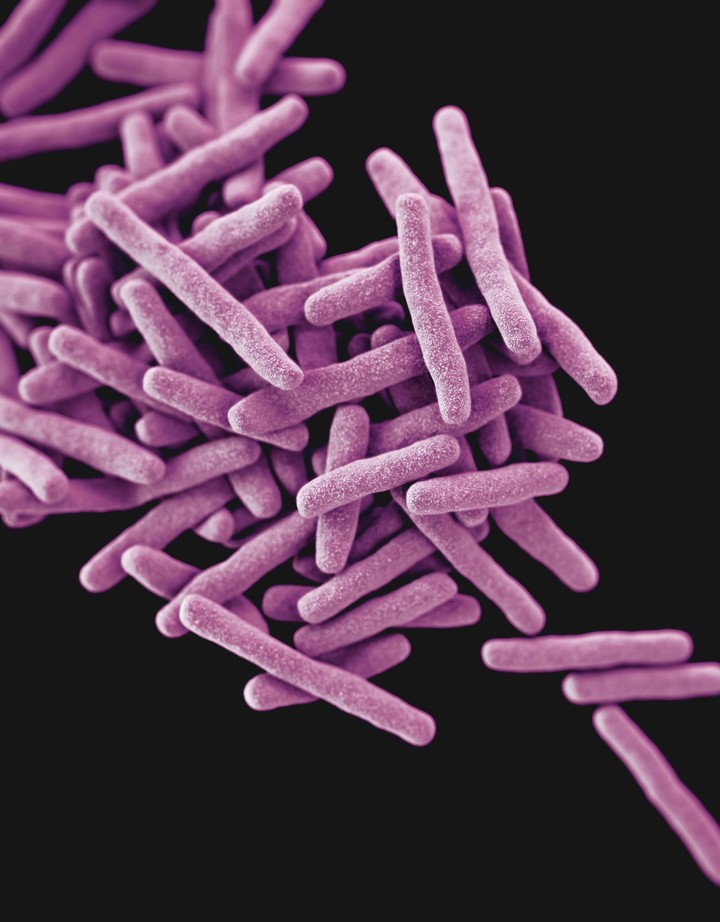
My postdoctoral research at UCSF has focused on the development of a Bayesian-supported framework for decision-making in adaptive Phase II trials; work performed in collaboration with the UNITE4TB public-private consortium to advance Phase II clinical trial design and identify novel tuberculosis (TB) therapeutics. TB is the leading cause of death from an infectious disease worldwide. Though treatable and curable, current therapeutic regimens have been ineffective in controlling the spread of TB. The identification of shorter, better tolerated regimens has been identified by the WHO and the NIH as a critical goal for addressing the unmet medical need in drug-susceptible TB populations.
In addition to developing the decision-making framework, I am in the process of designing a simulation study to evaluate the utility of a novel dose-finding methodology for optimal duration-finding for TB therapeutics. Though decreasing the required duration of treatment is a critical goal to improving TB outcomes, the statistical field of identifying optimal treatment durations is still in its infancy. This work will directly inform the statistical analysis plan for the DRAMATIC Trial, designed to identify the shortest effective treatment for multi-drug resistant TB.
I am also working with Dr. Phillips and colleagues at Harvard University on work funded by the Bill & Melinda Gates Foundation to improve best practices in identifying statistical targets (“estimands”) and their estimation for clinical trials in TB. Since the publication of the ICH E9(R1) guidelines, researchers in clinical trials have provided practical and theoretical guidance as to how the `estimands' framework may work in practice. This has increased discussion around best practices for defining meaningful and specific targets for estimation. However, pragmatic guidance around estimand-aligned estimation strategies is still needed. I am performing a simulation study to evaluate the feasibility and robustness of estimand-aligned estimation strategies to varying intercurrent event settings, using TB as a foundational case study.
Statistical methods in this area include Bayesian analysis, simulation studies, MCP-Mod, missing data and causal inference approaches, as well as parametric and non-parametric survival models.